3 analytics misconceptions holding your business back (and how to overcome them)
It’s game on for digital transformation. Success in this hyper-digital world requires meeting market demand and exceeding customer expectations. And without the use of advanced analytics and AI initiatives to deliver predictive, guided insights, organizations will fall behind.
According to IDC, a whopping 83% of CEOs want their organizations to be more data-driven, and the top priority for 87% of CXOs is being an intelligent enterprise. Yet that urgency is often stymied by perceived—but often inaccurate—obstacles.
If you’re anything like the leaders we work with across industries and organizations, these three common misconceptions may be impeding your advanced analytics efforts. But don’t worry—our best practices will help you overcome them to get your analytics strategy off the ground.
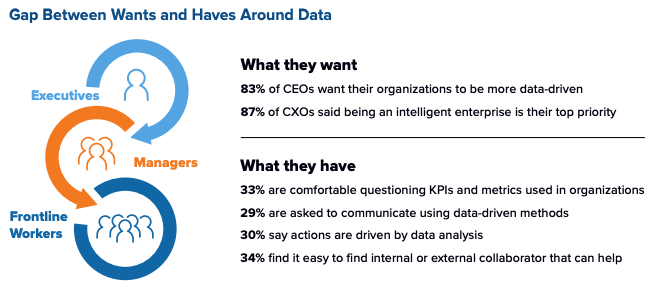
Building a Data Culture that prioritizes data-driven decision making takes more than just the right tools and technology. Though many leaders want to maximize data across the business, there’s a clear gap between what they want and what they have (source).
1. My data isn’t good enough.
If you think your data isn’t good enough—or you’re hearing this common refrain from others—I’m here to tell you otherwise. Your data is your data. After all, you’re already running your business on it.
Even if your data is dirty, siloed, or otherwise less than perfect, just get started. Don’t let perfectionism be the enemy of progress—take the first step toward artificial intelligence (AI) innovation.
Take your data lake, for instance. You don’t have to wait until your data repository is “done” to start an AI project because machine learning (ML) models won’t use every terabyte of data for each use case and prediction. The same goes for your CRM, which may be inconsistent or incomplete thanks to user-generated input data. Despite those gaps, you likely have enough data to create useful models to predict opportunities, win/loss, lead conversion, or churn.
For example, I recently worked with a wealth-management company embarking on a large-scale digital transformation. They had transitioned from an under-used, legacy CRM to Salesforce Financial Services Cloud, and even though they only had data from an outdated CRM, they were still able to use that data to build accurate predictive models to guide client engagement across the book of business. They gained powerful insights and experience to drive immediate business impact—specifically, to increase assets under management (AUM) and deepen client relationships. And over time, those insights—and data collection efforts—will continue to improve.
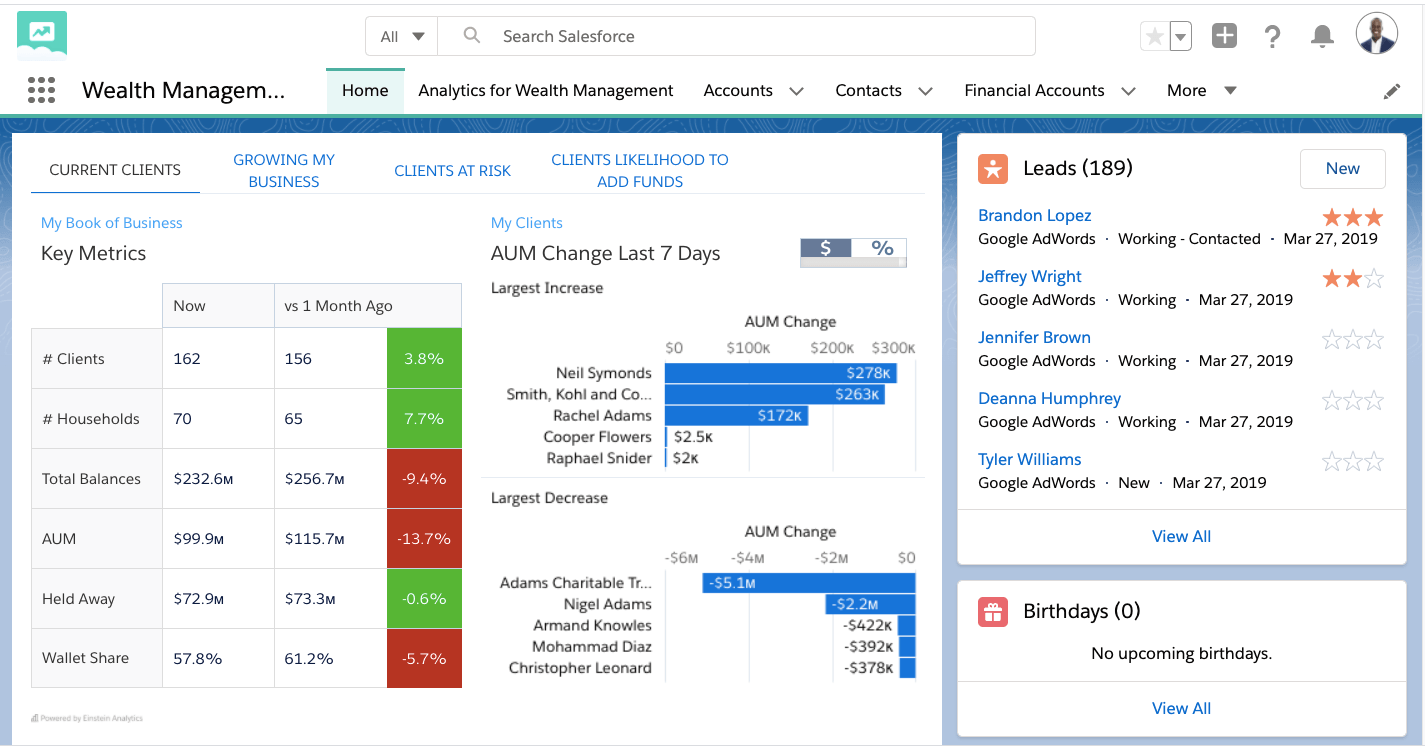
Even data from lackluster CRM systems can be used to build dashboards that offer impactful business insights.
Even if your data is dirty, siloed, or otherwise less than perfect, just get started. Don’t let perfectionism be the enemy of progress.
2. I don’t know where to start!
Advanced analytics projects are in high demand, but so much opportunity can also be overwhelming. Don’t let the blank screen paralyze you before you even begin. Use this framework to identify and prioritize the use cases with the greatest impact for your business.
Identify business needs.
Start with the strategic initiatives and KPIs of the business. Is it reducing churn and cross-selling products to increase revenue? Or customer lifetime value and other forms of brand engagement and measurement? Whatever it is, begin and end with your business goals.
Drill down to the needs of your customer-facing employees:
- How is their work connected to and impacting those business KPIs?
- What actions do they take? What decisions do they make?
- What makes those actions and decisions difficult? Where is the process broken or slow?
Examining these questions will help you pinpoint critical leverage points where you can use advanced analytics to remove friction and use predictions, insights, and recommendations to drive behavior and decisions at scale.
Ask: Am I addressing a million-dollar problem?
Does the project have the potential to increase conversions by 20%? Increase win rates by 30%? Get real about the expected impact—or lack thereof. You’ve hit gold when you land on that “million-dollar impact” that the business just can’t ignore.
Iterate, iterate, iterate
Balance the chase for that critical, business-changing problem by testing other use cases simultaneously. Take a rapid, iterative approach to quickly test results, abandon use cases that don’t pan out, and redirect efforts to impactful solutions.
You can’t just build models. You need to deliver simple, contextual, and trusted insights, so people actually use them.
3. If I build it, will they come?
When we talk about "you" building it, we're talking about you: the business leader. That's right, you don't always need a data science team to deliver on the promise of advanced analytics. Data science teams typically focus on coding models and model accuracy, not system integration and user experience. This disconnect makes it difficult to get customer-facing sales and service reps to use the insights you’re delivering.
If you want your analytics efforts to impact the quality of decision making and business ROI, you can’t just build models. You need to deliver simple, contextual, and trusted insights so people actually use them. Here’s how:
- Bring the insight to the user. Don't make them search for insights or “do analytics.” Put insights within the context of the business process and job at hand.
- Explainability and transparency are key. Is it clear how an insight was reached or a recommendation made? Is it easy to understand and take action, using language that is natural to the users consuming the insights?
- First impressions count. If a user has a validated experience with an impactful prediction, they’ll use it again. The same is true for a negative experience. Establish a baseline, so you can measure and celebrate ROI and business impact.
- ML is a full-team sport. Get stakeholder alignment early on. Have the business and data stewards guide key use- cases and KPIs.
- Governance is mandatory. Integrated governance builds trust and confidence, providing the right data to the right people at the right time, while maintaining your security and compliance standards.
- Ethics aren’t optional. Responsible AI use is a core part of your value proposition that must be communicated to your users and customers—and embodied in your technology tools.
Take the first step
Just get started. Now is the time to gain traction on your advanced analytics projects. Break through the misconceptions, and make your move. I think you’ll find it’s easier than you thought.