Whitepaper
Introducing Tableau Business Science
Tableau is bringing powerful data science capabilities to business people
Andrew Beers, CTO, Tableau
Executive summary
This paper introduces Tableau Business Science, a new class of AI-powered analytics that brings data science capabilities to business domain experts.
Using AI, machine learning, and other statistical methods to solve business problems has largely been the purview of data scientists. Many organizations have small data science teams focused on specific mission-critical and highly scalable problems. But, there are a large number of business decisions that rely on experience and knowledge in addition to data.
With Business Science, analysts and business users who understand the context of their data can train and deploy explainable machine learning models to problems that small, focused data science teams don’t have the time or resources to prioritize.
At Tableau, analysis has always been about letting people ask that next question, explore that next hypothesis, test that next idea. Now, we’re taking it further and helping more people elevate their human judgment with practical, ethical AI that brings predictions into their business problems today. This helps organizations make even faster, more confident decisions across their lines of business, while expanding their analytics use cases and deepening their understanding of their own data.
The barriers to making better decisions with AI
With the increasingly diverse sets of data that organizations are collecting, the analytics use cases to transform data into valuable insights are growing just as fast. Today, there are a wide range of tools and focused teams that specialize in finding data insights to inform decision-making, but organizations haven’t been able to strike the right balance between highly technical data experts and the business teams with experience and deep domain expertise. Not every company has a data science team or artificial intelligence (AI) solutions, and those who do often have a small, highly skilled team with a huge backlog of projects.
We often see that the business users and analysts with domain knowledge and proximity to business data don’t have the tools or technical skills to do advanced, statistical analysis or to manage machine learning (ML) projects themselves. They frequently rely on data scientists and ML practitioners to build and deploy custom models through a back-and-forth process of requirements gathering—a process that lacks agility and the ability to iterate quickly. By the end of the cycle, the data that the model was trained on is often stale and the process starts all over again. Meanwhile, business professionals are responsible for making critical decisions daily that don’t rise to the priority level for a centralized data science team.
Many of our customers see the value of applying data science, AI, and ML to more business problems, but feel constrained by resources and process. The common needs that we hear from organizations spanning different industries and sectors include the desires to:
- Reduce data exploration and prep work
- Empower analyst experts to deliver data science outputs, especially predictions, at lower costs
- Free data scientists’ time to allow focus on complex data engineering on mission-critical problems where exact precision is the most important consideration
- Increase the likelihood of producing successful models with more exploration of use cases by domain experts
- Extend, automate, and accelerate analysis for business groups and domain experts
- Reduce time and costs spent on deploying and integrating models
- Promote responsible use of data and AI with improved transparency and receive guidance on how to minimize and address bias
This is where we at Tableau see a huge opportunity to help organizations apply data science capabilities to more business problems, while minimizing the trade-offs between ultra precision and extreme control versus the time to insight—and the ability for people to take action on these insights while they’re still relevant. By bringing the core AI technology from Salesforce’s Einstein Discovery into Tableau, we’re introducing a new class of AI-powered analytics that seeks to democratize data science techniques and empower users to make faster decisions with greater confidence. And, we think this is a critical area for the market to continue innovating in.
Tableau democratized visual analytics and now we are doing the same for self-service AI. Business Science unlocks the vast potential of an army of professionals working with data every day. Taking these BI-savvy folks from descriptive analytics to advanced analytics, predictions, and recommendations means applying richer analysis to more use cases, faster and more collaboratively.
Introducing Tableau Business Science
What is Tableau Business Science?
Business Science is a new class of AI-powered analytics that allows people with domain expertise to make smarter decisions faster and with more confidence, recognizing that not all problems require exact precision at the expense of speed and business context. Business Science solutions are also rigorous and accurate, but enable decision makers to determine what they need for their use case with control and flexibility. A few examples of the way users can exercise control are the selection and shaping of input data, variable selection, and threshold setting. Business experts are empowered to enable a fully automated experience or have the choice to make guided changes in the model creation process. By equipping more people with governed, no-code AI—like predictions, what-if scenario planning, and guided model building, business teams can do more analysis themselves and produce more applicable, real-world-ready models.

Figure 1: People are empowered with transparency, control, and flexibility to make guided changes to threshold setting in Tableau CRM, for example, to get applicable predictions and insights.
Business Science democratizes data science capabilities and helps domain experts understand the key drivers of a model without having to learn traditional data science tools. With guided AI experiences in the hands of domain experts, teams can apply advanced analysis to more business problems and make important decisions faster and with more rigor, while still leaning into their human judgment. It’s not about fine-tuning super precise models, but guiding people closest to the problem in the right direction.
Who is Tableau Business Science for?
Business is inherently complicated and unpredictable, so domain experience and knowledge from people who understand the dynamics of their field is critical. Historical data, while a helpful input, is not always enough to answer how changing market conditions will impact your organization. The person who has the context of their field, and can adjust and respond on the fly, is the critical element for successfully navigating changing business environments. By enabling business professionals and data analysts to leverage the predictions and insights that come out of ML models—without having to learn Python, statistics, or how to tune parameters for an algorithm—you’ve started to grow your team of data-driven experts.
Business Science happens when you combine domain expertise and understanding with historical data and analytic insight. Typically, knowing the right question, and knowing what you are going to do with the answer, is more important than details like algorithm selection. Often these problems are more complicated than simple approve/reject decisions. Questions of resource allocation, prioritization, staffing, and logistics often require Business Science to get to the best data-driven decision.
We’ve observed countless scenarios where Business Science is the right approach that will result in the best outcome for the business:
- Marketing and sales can apply Business Science to lead scoring, opportunity scoring, predicting time to close, and many other CRM-related use cases that most data science teams can’t prioritize, but are highly valuable.
- Manufacturers and retailers can benefit from Business Science for supply chain distribution and optimization, forecasting consumer demand, or exploring scenarios for adding new products to their mix.
- Human resources might use Business Science to assess the likelihood of a candidate accepting an offer by analyzing historical patterns and factoring the recruiter’s knowledge of how they can adjust the mix of salary, equity, benefits, etc., based on the candidate’s values.
- Corporate real estate might apply Business Science to plan where to buy office space and explore the costs of moving people between buildings or office locations. Here, human judgment could help balance the right budget considerations with any “office politics” (i.e. implications that may come with moving certain individuals or teams).
Why Tableau Business Science?
AI projects need data expertise and domain expertise to succeed
Despite the fears and misconceptions that AI will replace people, there are too many business problems where the smartest machines are no match for human judgment. For example, a sales organization might use predictive modeling to determine the most profitable upsell/cross-sell opportunities. An algorithm can offer predictions about the likelihood that a customer will buy, but wouldn’t have the critical knowledge of someone managing the business relationship. An account executive would understand the customer’s goals and which products might help them achieve those goals, or may have learned through past experiences that a certain solution is unlikely to reach a consensus to buy.
These kinds of nuances require human judgment beyond what an algorithm alone can offer. Together, human expertise, judgment, and contextual awareness combined with the rigor, automation, and scalability of machine-generated insights drives better business outcomes. As another example, a retailer might want to explore which new products to add to its stores to increase profits in a certain region. A business professional understands how factors like supplier relationships, regional trends, and other impactful, qualitative implications could affect the decision—details that a machine might never understand or apply successfully.
Even with automation, the human should be able to understand and explain the outcomes. Most AI-based automation involves using mathematical algorithms for modeling and prediction and the recommendations should be continually tested by humans.
Organizations spend a lot of effort trying to recruit “unicorns” that have both data science and domain expertise, but people that fit this description are incredibly rare. There are a lot of mission-critical problems where data science techniques are the most important consideration, but Business Science brings many of these techniques to more people, so they too can leverage predictions in their analysis without requiring an advanced, technical degree.
Business Science is about matching the method and the expert to the problem. When data science teams take on new projects for the business, they often struggle from a lack of context and domain knowledge. Without the right context, data science teams spend a lot of time trying to identify the right data to address the problem, then curating, cleaning, and preparing it for analysis. As AI and ML rely heavily on data, this is fundamentally a data problem, so it’s important to have a good match between the problem they’re trying to solve and the right data—in the right shape—to do so.
The proximity of domain experts to the unique problems their organizations face, and their specialized knowledge about their line of business data, gives them an advantage in developing, validating, and deploying predictive models to be more timely, efficient, and impactful.
An iterative, revise-and-redeploy approach saves time, reduces costs, and elevates unique skills
Traditional data science and ML cycles can be long and the “last mile” is often the most challenging and resource-heavy in terms of time, effort, and cost. Deploying and integrating traditional custom models is complex, requiring statisticians and data scientists to make solutions consumable and actionable by end users. When time to market is a major factor, empowering people to create and iterate on predictive models in a matter of days and weeks—not months—maximizes resources and helps to deliver a better return on investment.
Many organizations struggle to scale their AI prototypes and pilots to full production and wider usage, and often underestimate the challenge of deploying and integrating AI with other systems. According to the 2020 Gartner AI in Organizations Survey, only 53% of prototypes are eventually deployed.
For business experts who need to lean on data and predictions to inform decision-making, it’s incredibly valuable to be able to control various aspects throughout the model-building process and have the appropriate confidence in their data relative to each use case. This is especially worthwhile when the model-building approach is iterative and focused on continuous improvement of prediction accuracy. Such an approach helps people take action while the data is still relevant. And sometimes, even attempting to build a model can cause a team to prove the data to the point that they’ve achieved some value.
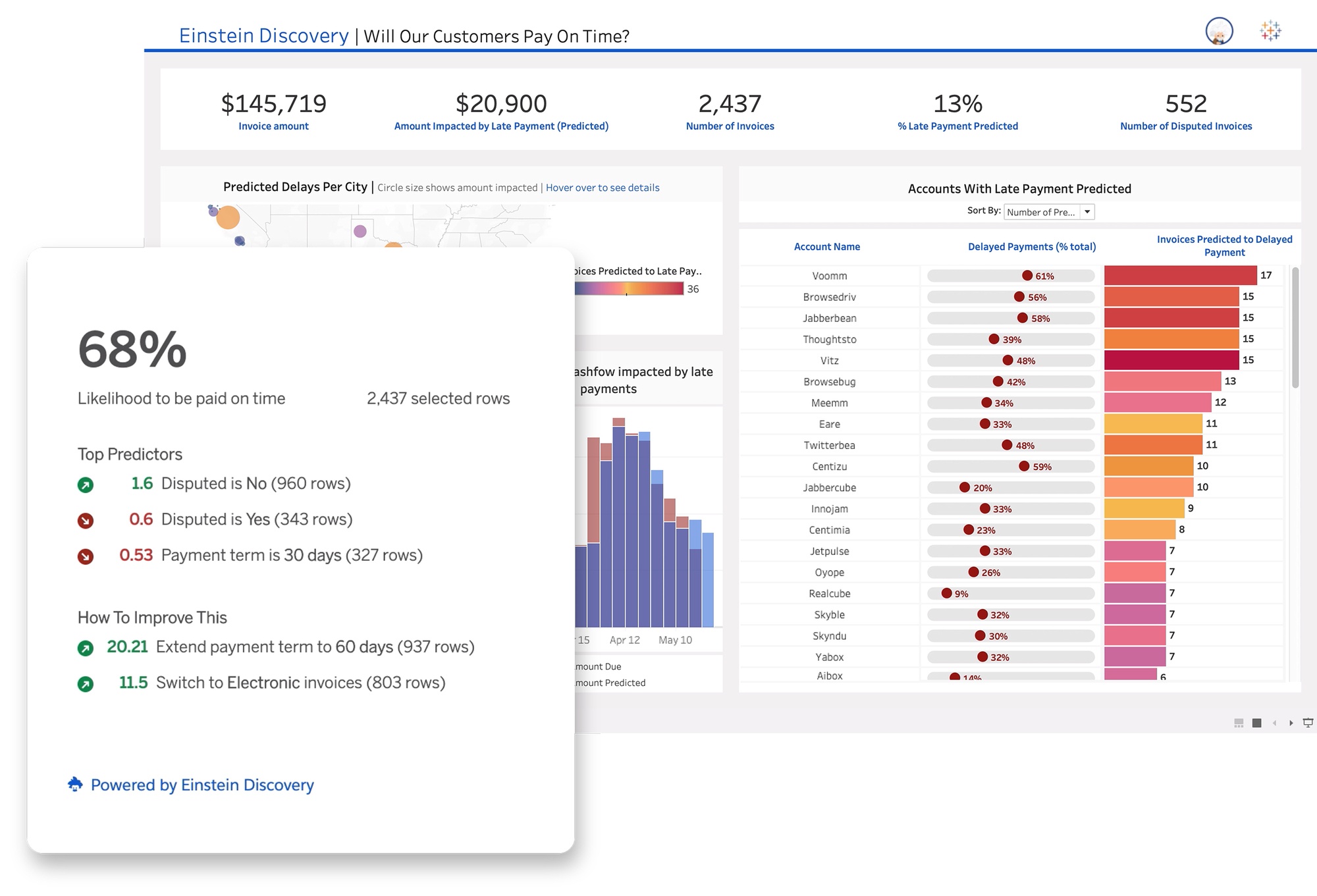
Figure 2: Integrated in the analytics workflow, Einstein Discovery offers dynamic predictions embedded in Tableau dashboards.
With Business Science, the objective is to move a KPI—not to perfect a model until it’s the most precise. By facilitating a more iterative, revise-and-redeploy process than that of traditional data science cycles, Business Science eliminates barriers for people with business context to quickly build models and apply predictions. More people can get value faster from these advanced analytical techniques and make smarter decisions when and where they need to—potentially leading to major cost savings or profits for a specific use case.
Think about assigning sales quotas: geographical assignments and targets change all the time. The same model for assigning quotas one year won’t work for the following year, because the inputs to their model are going to continuously evolve. And what if a regional sales lead vetoes the suggested model because they have a goal to do even more business in their geography? This makes the ability to quickly pivot or iterate on the model really valuable, and potentially result in new opportunities to generate more revenue in these sales territories.
By enabling analysts and business users with the ability to safely self-serve with machine learning, Business Science elevates the unique skills and domain expertise of more people. It also reinforces the integral role data science teams play by freeing them up to focus efforts on large-scale, mission-critical projects. This class of AI-powered solutions can also create an experimental environment for analysts and advanced business users to explore new use cases that aren’t priorities for data science teams. With more people examining larger numbers of use cases and analyzing drivers of potential outcomes, the likelihood of delivering a successful model increases. Domain experts are empowered to build no-code, ML-driven models for their needs, producing excellent, business-specific analytics that don’t require the level of depth that data professionals go into.
It’s important to note that while Business Science solutions can help address the skills gap between analysts and data scientists, it is not a replacement for them. Data science professionals will continue to provide custom models, statistical analysis, etc., but more often, they will partner with business experts to validate the data being used in ML-powered models. This increased cross-team collaboration remains critical to the success and performance of these solutions.
Democratizing data science responsibly requires guidance to identify and mitigate bias and unethical use
Expanding AI-powered analytics to more people has many benefits, but also some potential dangers. Bias is inherent in data and technologies, and unless mitigated, can perpetuate bias through predictions and recommendations—even causing harm. Facial recognition technology is a well-known example of a confluence of failure points, where biased training data, technology, and predictions have created harmful outcomes for communities of color. (This is one of the reasons why we don’t allow facial recognition technology in our acceptable use policy.)
The average business professional isn’t necessarily thinking about these things in their analysis. As a start, robust model documentation can provide more transparency behind predictions and recommendations and make things easier to trace. Understanding what contributed to the prediction, what columns in the data, and where the drivers are can help identify potential bias in your data sets and models.
There is no automated or blanket solution to ensure ethical use of data and AI—you really have to know the data yourself. But a responsibility we owe our customers is to put guardrails in our technology where we can flag the potential for harm: to help customers not persist the bias that exists in their data into their predictions and then apply that to real data as it’s coming in.
Further guidance might look like built-in bias detection, model monitoring, and natural language explanations of the variables influencing predictions, helping people create more ethical models. With Einstein Discovery, the product asks users to select variables and sensitive fields that could possibly include bias—like race, age, marital status, and residence location. Through proxy detection, it will also look for correlated fields in the data set, where there is potential for bias if one field, but not the other, were removed. Another important metric is disparate impact: for example, seeing if the outcome of the model differs across genders or different zip codes. For many regulated industries, this is one metric used to hold machine learning models accountable.
Democratizing AI and ML comes with important considerations and responsibilities
This is an emerging field, so it’s unclear how the landscape will change as new technologies and use cases are discovered, and as ethics standards and regulations become more commonplace. AI technology will further be shaped by forthcoming regulations from governing bodies in the European Union, and as more state and federal governments in the US implement new artificial intelligence controls. Given the evolving landscape and level of disruption seen in the past year, organizations are being put to the test: Digital transformation is no longer on the horizon, but is here. Enterprises are figuring out ways to manage the current influx of data and AI innovation and its effective applications—including how to use AI to be more agile and resilient.
Business Science enables greater access to machine learning, but the responsibility lies with organizations to manage the development and use of AI securely and ethically. Tableau and Salesforce provide the platform and guidance for individuals and organizations to make more informed and responsible decisions. The customer understands their data better—and the business context in which AI and ML solutions are to be applied. We believe that combining powerful AI and ML solutions with human expertise is the most effective and powerful way to balance the right levels of cross-team collaboration, ethical AI use and application, and rapid iteration. This human-centered approach also helps predictive models, scenario planning, simulation, and other data science techniques be more effective and successful in their use.
As we continue to innovate, and as our customers tackle existing challenges and discover new ones, Business Science best practices will emerge. We’ve identified important considerations as organizations adopt and develop Business Science solutions, aligned with our values. To find the right balance across the factors of collaboration, rapid iteration, and ethical use, we feel it’s critical that organizations:
- Define and agree on roles and processes
- Establish methods for successful collaboration
- Build in human touchpoints for ethical use and development of AI
Define and agree on roles and processes
Domain experts and data scientists will have to adjust to this new environment of self-service AI. When implementing Business Science solutions, it’s important for analysts, business users, and data science teams to define and agree on roles, what work needs to be done, what new processes may be required for smooth and productive exchanges, what level of involvement and validation is anticipated from each group, and other considerations. Like with any new tools or processes, there will be an adjustment period where recently-defined roles and processes are tested. Maintaining open dialogues will be critical to setting all teams up for success.
Establish methods for successful collaboration
As a part of maintaining dialogues across teams, it’s imperative that methods for continued communication are established. Channels may already exist, but standardizing and making a habit of actually using these channels will require conscious effort during the implementation of ML solutions to a broader group of people. And setting up a regular cadence of opportunities to ask questions, consult, validate, share honest feedback, etc. can make cross-team collaboration with AI-powered analytics successful.
Build in human touchpoints for ethical development and use of AI
Technology-based controls against bias are useful and support more people getting insights and predictions for smarter decision-making, but shouldn’t be the only auditing method to rely on. Be intentional about building in touchpoints for a human to review every step of the way. Analysts and business users have the domain expertise to understand the data in context. They may also foresee the potential effects when sensitive data isn’t handled ethically, but this is only possible with a diverse employee base: people who are able to identify gaps or discriminatory uses in the data, and can speak for underrepresented populations who are most negatively affected by biased AI systems. Machine learning and human expertise—combined with the necessary tools, human review, transparency, and bias monitoring—help organizations apply AI solutions securely and ethically.
If you see that your data is already biased and your model has the potential for causing harm, you should not deploy the model or automate any decision-making. Addressing bias in your data is critical across multiple factors. Not only can bias result in harm, bias in your data also means that your data has errors, which subsequently leads to mistakes in your models, their predictions, and related decisions. If your organization lacks an internal ethics group or has concerns about how to address the bias, partner with third-party experts to help you thoroughly examine and mitigate bias in your data or models. Their investigations can reveal potential consequences of letting bias go unchecked. Your data science teams can help support the work done by these external AI auditors and consultants.
At Tableau and Salesforce, we’re committed to delivering tools to our customers, partners, and employees, for developing and using AI responsibly, accurately, and ethically. Read more about our commitment to AI ethics from the Salesforce Office of Ethical and Humane Use. To help remove bias from your data and algorithms for more ethical AI systems at your company, complete the Salesforce Trailhead module, “Responsible Creation of Artificial Intelligence."
Conclusion
The demand for data skills is only growing, and with it, tremendous opportunity to help people and organizations learn and solve problems more effectively. While some approaches may focus on developing or implementing completely automated AI solutions, this limited approach will likely fail to deliver the value it’s intended to provide. Discounting and removing human ingenuity, contextual awareness, and expertise when democratizing AI and data science techniques isn’t just counterproductive, it’s irresponsible.
Tableau has always believed in the power of human potential. Unleashing human potential is a part of Tableau DNA, having been built into our industry-leading visual analytics platform. Now, with Tableau Business Science, this new class of innovation uses AI and ML to continue our tradition of empowering people with the right tools and functionality to help them ask and answer questions, uncover insights, and solve problems. With the introduction of Einstein Discovery in Tableau, a Business Science solution, we’re safely putting machine learning into the hands of more people, democratizing powerful data science techniques to help people make better, faster decisions.
Additional resources