Soluções
A Tableau está empenhada em uma coisa: ajudar as pessoas a ver e a entender seus dados. As organizações em todo o mundo e em todos os setores e departamentos, desde organizações sem fins lucrativos a empresas globais, estão capacitando seus funcionários com dados. Independentemente de sua função, as pessoas e as equipes estão usando o Tableau para descobrir novas informações e oportunidades e criar uma cultura de tomada de decisões impulsionadas por dados como nunca houve antes. Saiba mais sobre como organizações como a sua estão usando a plataforma do Tableau para soluções de visualização de dados e business intelligence.
O Tableau transforma a maneira como as organizações usam os dados
Como diferentes setores usam o Tableau para business intelligence
Independentemente do setor, empresa ou função, o Tableau pode dar vida aos dados por meio de análise avançada e interativa. Organizações em todo o mundo usam o Tableau para tomar decisões impulsionadas por dados de maneira rápida e confiável.

Saúde
Organizações nos setores de saúde e ciências da vida reúnem dados de saúde pública e de pacientes para avaliar e alocar recursos de programas que aprimoram o atendimento dos pacientes e salvam vidas.

Educação
Escolas de todos os níveis de ensino podem unir análise sofisticada e educação, usando dados para identificar métricas que indiquem o sucesso dos cursos e dos estudantes.

Finanças
O Tableau ajuda a substituir relatórios estáticos e manuais por painéis interativos, estejam os dados no local ou na nuvem, para serviços bancários e gerenciamento de fundos e até mesmo seguros, entre outros.

Setor público
Agências governamentais, associações e organizações sem fins lucrativos usam o Tableau para monitorar o impacto de seus programas e aumentar a transparência com visualizações interativas que tornam os dados mais fáceis de entender para todos.

"A melhor parte do Tableau tem sido o impacto que vemos nas comunidades que atendemos. Os dados impulsionam discussões sobre para onde queremos ir, nossas expectativas, nossas esperanças e os impactos que esperamos ter."
Diversos departamentos e funções usam o Tableau para trabalhar juntos
O Tableau oferece para toda a sua organização uma velocidade transformadora rumo às informações e à autossuficiência por meio de análises interativas e visuais. Alinhe as equipes quanto aos objetivos e às expectativas e inove ao responder rapidamente às necessidades dos clientes.


Suporte e serviços
A análise oferece aos departamentos de suporte e serviços a visibilidade dos clientes e das operações necessárias para otimizar sua experiência e aumentar sua satisfação e a lealdade.

Finanças
Com o Tableau, os departamentos financeiros podem ir além das planilhas e dos processos manuais para analisar o fluxo de caixa, viagens e gastos e muito mais com os painéis interativos.
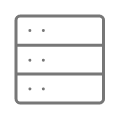
TI
O Tableau pode oferecer à gerência de TI um panorama completo dos negócios para ajudar a administrar, monitorar e manter todos os sistemas da organização, com recursos robustos de integração.
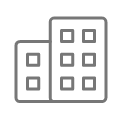
Liderança
Executivos com qualquer nível de habilidade técnica podem entender os dados e fazer as perguntas certas, com visualizações de dados que oferecem informações acionáveis.
O Tableau funciona perfeitamente com as principais tecnologias em todos os seus pipelines de dados.
O Tableau tem uma ampla rede de parceiros com os melhores nomes nos setores de nuvem, preparação e gerenciamento de dados, ciência de dados e mais. Nossas integrações garantem uma solução avançada de análise moderna e completa que se adapta a seus investimentos tecnológicos atuais.




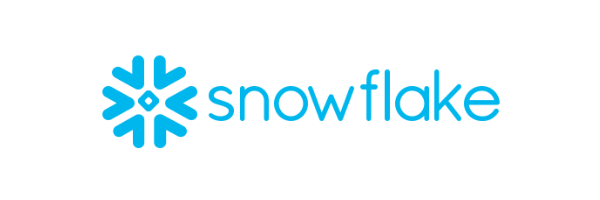


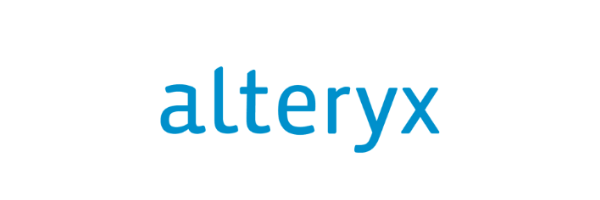



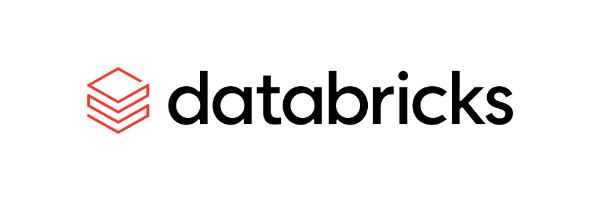
O Tableau Blueprint ajuda você a criar uma cultura impulsionada por dados
Com análise intuitiva para todos, o Tableau ajuda as organizações a desenvolver uma cultura de dados que valorize e priorize a tomada de decisões bem-embasadas. Sabemos que é preciso mais do que a tecnologia certa para realizar uma mudança duradoura. O Tableau Blueprint combina nossos conhecimentos e as práticas recomendadas dos clientes em uma metodologia passo a passo para criar uma organização impulsionada por dados.